High-Frequency Trading (HFT): Definition, Origin, Strategies, Return, Regulations
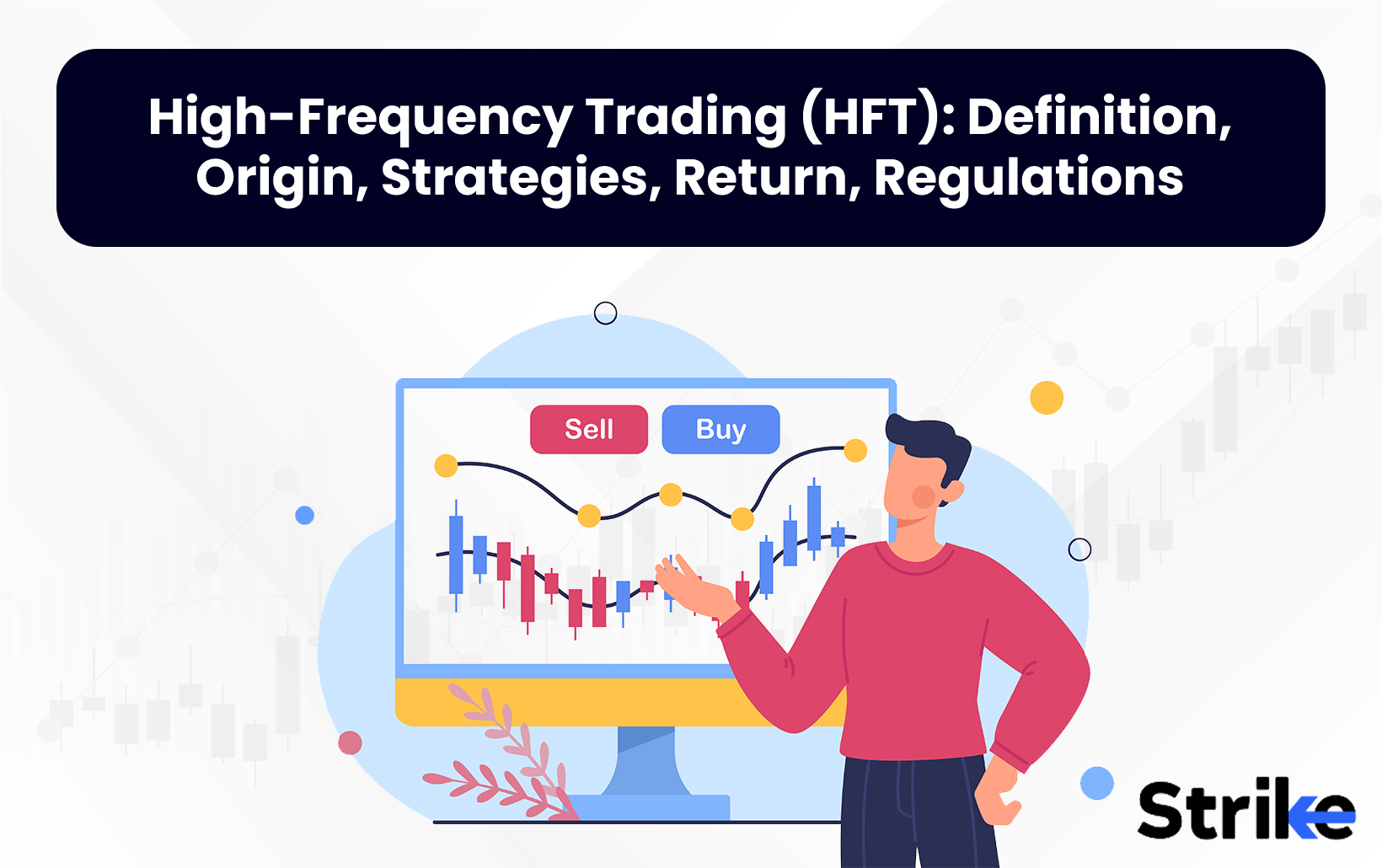
High-frequency trading (HFT) is a type of automated trading that utilizes powerful computers and algorithms to transact a large number of orders at extremely high speeds. HFT originated in the late 20th century as computerized trading technology advanced and financial markets grew in complexity. The origin of HFT was a response to develop systems that could capitalize on short-lived opportunities through rapid transaction execution. HFT plays an important role in modern markets as it contributes to liquidity and pricing efficiency. One main advantage of HFT is its ability to quickly capitalize on momentary price anomalies. However, one limitation is that it can exacerbate volatility during periods of high market stress due to the massive order flows generated by HFT algorithms. Understanding both the origins and strategies employed in HFT helps market participants better navigate todayâs highly electronic financial system.
What is High-Frequency Trading(HFT)?
High-frequency trading (HFT) is a type of algorithmic trading that involves executing a large number of orders in fractions of a second. High-frequency trading firms use powerful computers and advanced algorithms to analyze market data and place trades at extremely high speeds. The goal is to identify trading opportunities, like arbitrage opportunities, and execute orders just before the rest of the market reacts.
HFT has become very prevalent in the stock market over the last couple of decades. Certain estimates say HFT accounts for over half of all trades in US equity markets. Proponents argue that HFT provides liquidity and tightens bid-ask spreads. However, critics say it gives firms with the fastest systems an unfair advantage and increases volatility. Flash crashes like the one in 2010 have been partially attributed to HFT. Regulators continue to debate if additional oversight or regulations are needed. However, HFT will likely remain an influential force in stock trading given the competitive advantages it provides firms willing to invest in the infrastructure and technology required.
What is the origin of high-frequency trading?
High-frequency trading (HFT) emerged in the late 1990s as technological advances allowed for ever-faster trade execution times. In the beginnings of electronic trading in the stock market, trades were measured in minutes or seconds. This gradually improved to trade execution times measured in milliseconds and then microseconds. As trade speeds accelerated, a new type of proprietary trading firm arose that used algorithms to analyze market data and place trades at rapid speeds, aiming to capture small profits per trade.
The earliest high-frequency trading firms included Getco LLC, founded in 1999, and Tradebot Systems, founded in 1999. These firms used strategies like market making and arbitrage to profit off tiny price discrepancies in stocks. Early HFT focused heavily on the NASDAQ stock exchange, which was one of the first exchanges to go fully electronic in 1983. This allowed algorithmic trading firms to send orders directly to the exchange via computer systems and receive confirmations of trades executed in milliseconds.
In the 2000s, high-frequency trading expanded to other asset classes beyond equities. Futures, foreign exchange, and fixed-income markets saw a rise in HFT as exchanges moved to electronic trading systems. More and more proprietary trading firms adopted high-frequency strategies as a way to gain a competitive edge in the markets. Estimates suggest HFT grew to account for over 70% of trading volume on US stock exchanges by 2009. Firms made massive investments in technology like co-located servers and fiber optic networks to shave milliseconds off latency.
In 1998, the SEC authorized electronic exchanges to compete with NYSE and NASDAQ. This led to around a dozen electronic communication networks (ECNs) that competed for HFT order flow. In 2007, the Regulation National Market System (or Reg NMS) was implemented, which protected orders on electronic exchanges from being traded through other exchanges. This gave advantages to HFT strategies.
The rapid rise of high-frequency trading came into the public spotlight in the May 6, 2010, Flash Crash. On that day, the Dow Jones Industrial Average plunged over 600 points in minutes before rebounding almost as quickly. An SEC investigation found that HFT strategies exacerbated the decline by rapidly pulling liquidity from the market. This highlighted the risks created by the stock marketâs growing reliance on high-frequency traders.
In the 2010s, HFT faced increased scrutiny and criticism from regulators and the public. Countries in Europe moved to ban certain HFT practices. In the US, the SEC looked at ways to monitor HFT firms and make sure their systems did not malfunction. Also in 2010, author Michael Lewis published Flash Boys, which criticized HFT for using speed advantages to profit at the expense of other investors. The book further turned public sentiment against unregulated HFT practices.
How does high-frequency trading work?
High-frequency trading (HFT) works by using sophisticated algorithms and high-speed connections to rapidly trade securities in the financial markets. HFT firms utilize advanced technologies and infrastructure to execute large numbers of orders at extremely high speeds measured in milliseconds, microseconds, or even nanoseconds.
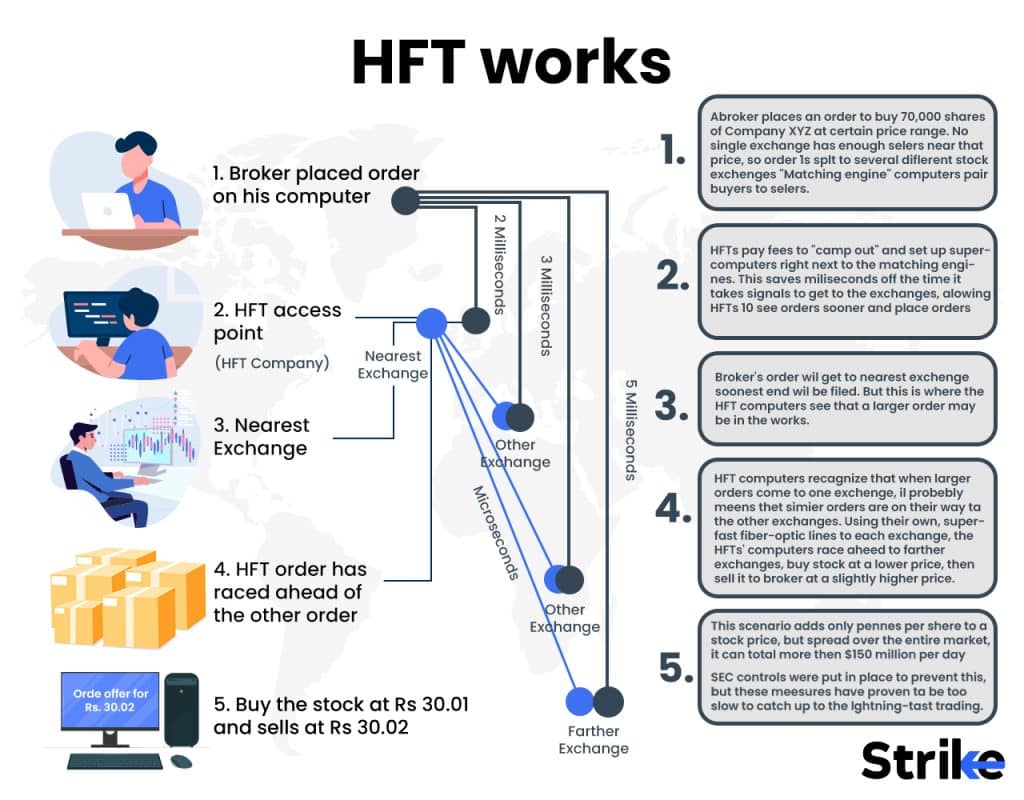
At the core of HFT are complex algorithms that analyze market data and price trends to identify trading opportunities. These algorithms are programmed to detect even the smallest arbitrage opportunities or instances of market inefficiency. For example, the algorithms will quickly detect this and initiate trades accordingly if a stock price becomes even slightly misaligned with its underlying value or compared to related securities. The speed of HFT algorithms gives them an advantage over human traders in identifying and capitalizing on momentary pricing discrepancies. The algorithms are designed to divide trading decisions into precise rules and automatically execute orders once certain parameters are met. This eliminates emotional biases and delays. While human discretion improves trading outcomes in certain instances, the split-second speed of automated algorithms allows HFT firms to benefit from fleeting opportunities faster than any trader could manually.
HFT systems require state-of-the-art technological infrastructure to achieve the processing power and connection speeds necessary to capitalize on ephemeral trading opportunities. This includes colocation services and individual server racks at securities exchanges that allow proximity to the system and faster trade execution. It also includes direct data feed connections that transmit market data directly from the exchange rather than through third-party aggregators, reducing latency. HFT firms also utilize microwave and laser transmission technologies to shave nanoseconds off communication times between trading centers. They invest heavily in field-programmable gate array (FPGA) processors, which are optimized for algorithmic trading applications much more so than commercial PC processors. The advanced infrastructure allows HFT systems to react to market developments and submit orders in a matter of microseconds. Even these increments of time are crucially important due to the short-lived nature of pricing inefficiencies. The expensive technological requirements act as barriers to entry in high-frequency trading.
How do I start HFT trading?
Launching a high-frequency trading (HFT) firm requires extensive technological infrastructure, trading strategies, adequate capital, regulatory compliance, and the ability to quickly execute orders in milliseconds. Before getting started, it is important to thoroughly research HFT and develop a detailed business plan and trading approach.
The most critical component of an HFT firm is a low-latency trading system. This allows the firm to rapidly send, execute, and process trades in fractions of a second. To minimize network latency, servers need colocation at data centers near exchange servers. Established data center providers offer colocation services. Top exchanges like NYSE and NASDAQ also have on-site data centers. The firmâs developers will build proprietary trading algorithms optimized for speed on the co-located servers.
Strategies that HFT firms implement include market-making, arbitrage, and liquidity detection algorithms to capitalize on momentary pricing discrepancies. Statistical arbitrage and pairing algorithms are also common. Machine learning and AI help uncover complex signals and patterns to inform trading decisions. Firms should specialize in a niche trading strategy suited to specific market microstructures. This helps gain an edge over other HFT players.
To scale up operations, a HFT firm needs significant capital. A high six-figure investment is generally minimal for infrastructure like hardware, data feeds, and colocation. Ongoing expenses like data center rack space rack up, too. Many firms are founded by former exchange traders or tech experts and start with their own capital. Venture funding or loans provide additional startup funding.
Robust compliance and risk management are mandatory. All electronic trading firms must register as broker-dealers with FINRA and the SEC. Regular reporting, capital requirements, trading records, and other regulations must be followed to avoid hefty fines. Compliance staff help monitor trading systems and ensure regulatory policies are maintained as the firm scales up.
A low-latency order routing network is required to enter orders on exchanges in microseconds. Direct market access (DMA) order routing and smart order routers are common technologies employed. Network connectivity through fiber optic lines, microwaves, and other means helps minimize communication delays. Redundancies are important in case of any network outages.
To build and monitor the trading infrastructure, the firm will need to hire expert personnel. Technical talents like programmers, network engineers, and systems architects design the architecture. Quant developers code complex trading algorithms and pricing models. Traders fine-tune the live trading strategies. Other key hires include management, compliance, operations, and sales staff.
Once the trading system is built, it undergoes rigorous testing. Load testing confirms capacity holds under high volumes. Latency statistics are analyzed to find bottlenecks. Algorithms are back-tested on historical data. Security tests are performed. Exchange certification is sometimes required before going live. After thorough testing, the firm started trading cautiously with small volumes to confirm that the systems worked as expected.
What are the popular strategies for high-frequency trading?
The popular strategies used for high-frequency trading are market making, quota stuffing, ticker tape trading, statistical arbitrage, index arbitrage, news-based trading, and low latency trading. These strategies aim to capitalize on short-term market inefficiencies and price fluctuations.
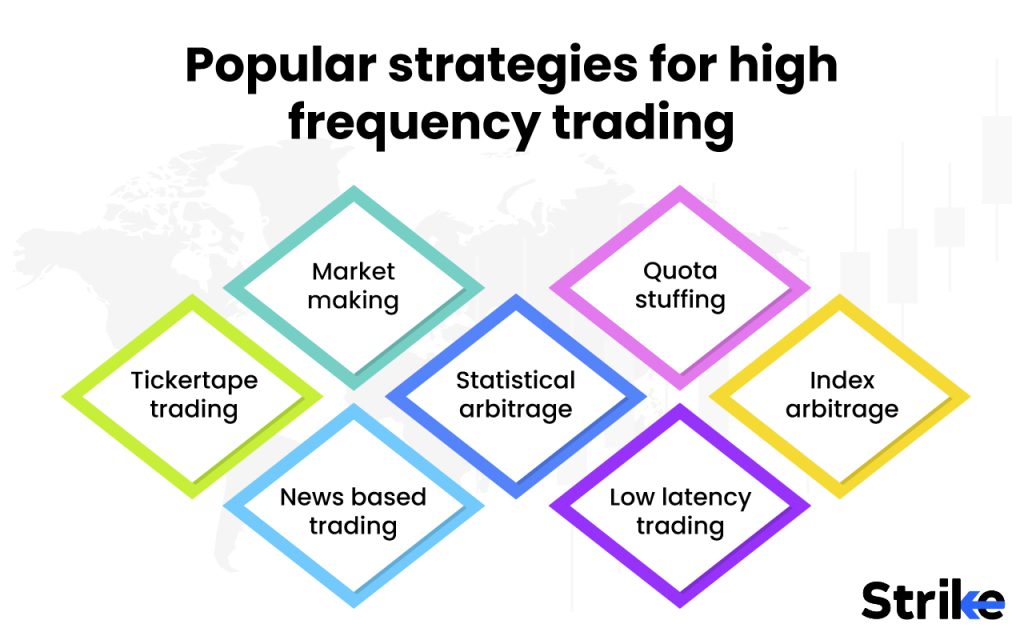
1.Market making
Market making involves continuously posting limit orders to buy and sell securities, aiming to profit from the bid-ask spread. High-frequency trading (HFT) firms use low-latency infrastructure and machine learning algorithms to update quotes rapidly based on market conditions. The goal is to maximize spread capture over time while ending each day flat. Speed is critical, but predictive modeling is the key advantage. Market making thrives during volatile markets with wider spreads but operates in any liquid product.
HFT market-making focuses on the most liquid securities like large-cap stocks and ETFs. Algorithms input countless data points to forecast expected trading activity and optimize quoting strategies. Historical trade data trains the models to adapt quoting to changing conditions. Colocation, microwave networks, and specialized hardware like GPUs reduce latency. However, predictive algorithms drive the quality of the quotes.
Market makers provide liquidity and tighten spreads, especially in thinly traded securities. They compete with other liquidity providers. Exchanges sometimes provide incentives like maker-taker pricing. For active stocks, competition is fierce, and ultra-low latency is critical. Strategies rely on speed to access quotes.
After the âflash crash,â regulations introduced quote minimum life and âspeed bumpsâ in market data. However, market-making remains an accepted HFT strategy. While reducing costs for investors, fast market-making raises fairness concerns. Exchanges must balance incentives so markets operate fairly.
With prudent regulations, high-frequency market making adds value through enhanced liquidity, tighter spreads, and reduced volatility. Though transaction costs are lowered for all, giving HFT firms an edge raises concerns that exchanges must address. By continuously posting competitive quotes, market makers improve readability, especially for low-volume securities.
2. Quota stuffing
Quota stuffing is an unethical and illegal practice in the stock market where a trader floods the market with non-bona fide orders to give the illusion of activity and interest in a particular stock. The goal of quota stuffing is to artificially drive up demand and prices for the stock in which the trader holds a position.
Quota stuffing works by exploiting the limit order book system used by stock exchanges. The limit order book shows all outstanding buy and sell orders for stock, organized by price level. Traders look to the order book for indications of supply and demand imbalances to inform their trading.
Quota stuffing is the practice of a trader entering a high number of buy or sell orders without intending for them to be filled. For instance, a trader might place purchase orders totaling 100,000 shares at prices between $50 and $55 if they have a long position in stock XYZ, which is now trading at $50 per share. This influx of buy orders creates the false appearance of strong buying interest in XYZ. Other traders seeing all of these pending buy orders in the order book are sometimes misled into thinking there is upward price momentum building for XYZ. In reality, the trader engaging in quota stuffing has no intention of buying those 100,000 shares â they are just spoofing orders to mislead the rest of the market.
Once the desired price movement happens, the trader cancels their fake orders before they are executed. For example, after entering the 100,000 shares in buy orders, the price of XYZ stock moves up to $53 per share. The quota-stuffing trader then cancels their open buy orders, locking in a $3 per share gain on their existing long position. The buy orders were never meant to be filled in the first place â they just served to artificially inflate demand.
Quota stuffing is considered illegal market manipulation and is prohibited under securities laws and exchange regulations. Regulators like the Securities and Exchange Commission (SEC) look for patterns of order spoofing and bring enforcement actions against traders engaging in quota stuffing. Exchanges also monitor for abnormal order activity and take disciplinary action like fines, trading bans, or loss of exchange memberships.
Traders engage in various techniques to disguise their quota-stuffing practices and avoid detection by regulators and exchanges. These include spreading stuffed orders across trading venues to prevent concentrated activity, using algorithms to quickly enter and cancel orders to mimic high-frequency strategies, limiting order sizes to normal market lots, focusing stuffing at key liquidity points like round number prices, and executing a small portion of orders to maintain a low cancel-to-execute ratio that avoids triggering automated surveillance systems. Despite these efforts at deception, regulators continue to monitor patterns of manipulation and punish those engaged in unethical quota stuffing.
3. Ticker Tape trading
Ticker tape trading involves algorithms that monitor news and market data to trade on significant events before they are fully priced into securities. News wires, disclosures, economic data, and other sources provide valuable information. The key is detecting and reacting to events faster than human traders using natural language processing and machine learning. Trades are executed within milliseconds or microseconds of major announcements that will impact prices. Strategies must filter noise and focus on events likely to move markets.
Low latency networks and co-located servers allow for the near-instantaneous capture, analysis, and trading of information. Natural language processing handles unstructured data like press releases or social media. Machines donât get caught up in the emotions around news events â algorithms capitalize on predictable short-term momentum. Major announcements from central banks and companies offer trading opportunities. Earnings reports, mergers, clinical trials, regulatory rulings, and geopolitics sometimes trigger trades.
Restrictions were introduced after 2010âs âflash crashâ to prevent volatility around news. However, valuable signals still exist in textual data. Identifying and reacting seconds faster than human perception provides an edge. The space between release and dissemination offers opportunity. Preprogrammed logic reacts to keywords, semantic analysis, and sentiment changes.
Sometimes, certain strategies assume announcements will cause momentum. Others try to predict magnitude based on historical data. Advanced machine learning models incorporate risk analysis for sharper forecasts. For anticipated events, much of the price movement often occurs pre-release during speculation rather than after. Algorithms decode early signals.
Looking ahead, AI advances will allow a more powerful contextual analysis of events. However, interpretable models are needed rather than black boxes. Controls against manipulation will preserve stability around news events. Ticker tape trading has evolved from paper ribbons to complex algorithms capitalizing on valuable information faster than humanly possible.
4. Statistical arbitrage
Statistical arbitrage refers to exploiting short-term statistical inefficiencies in market prices across securities or exchanges to earn riskless profits. Statistical arbitrage aims to profit from temporary mispricings between historically correlated securities. Algorithms monitor hundreds or thousands of instruments across markets to find co-dependent relationships. Trades capture the reversion when spreads diverge past historical norms. Machine learning uncovers complex statistical interrelationships between securities in different sectors and asset classes. Trading signals come from deviations in pricing relationships rather than directional views. Portfolios are constructed to be market-neutral. Position sizes scale with the arbitrage opportunity when spreads widen.
Microwave networks, fiber optics, and colocation provide the low-latency feeds and fast order execution required. Speed allows profits before slower traders compete for mispricings away. Statistical arb evolved from simple pair trading to sophisticated multidimensional strategies leveraging computing power. The massive scale of data analysis and rapid trading distinguish it from traditional quant funds. Millisecond speed and frequent rebalancing boost efficiency.
The holding period depends on the relationship dynamics, cause of distortion, and degree of displacement. Sometimes, certain anomalies are corrected quickly; others take more time. Algorithms optimize trade timing based on past behavior and liquidity constraints. Risk comes from the spread converging, not overall market exposure. Limits prevent excessive risk. Intraday rebalancing responds to new data.
For equities, related stocks, ADRs, ETFs, indices, and options offer numerous pair trading possibilities. In other assets, corporate and sovereign bond yield spreads present opportunities. Currency, commodity, and volatility relationships are targeted across the globally integrated market.
Statistical arbitrage continues to evolve as a profitable strategy for sophisticated high-frequency traders. While adding market efficiency by correcting anomalies, regulators watch that strategies do not manipulate markets. With oversight, stat arb fosters price discovery, liquidity, and relationships grounded in fundamental value.
5. Index arbitrage
Index arbitrage involves high-frequency traders simultaneously buying and selling the components of an index and the index itself to profit from temporary pricing inefficiencies between them. Index arbitrage aims to profit from price discrepancies between an index fund or ETF and its underlying basket of stocks. Opportunities arise around index rebalances when passive funds must buy and sell to match new weights. This causes quotes to deviate from net asset value. Arbitrageurs detect this activity using models. They trade the overvalued stock against the lagging ETF to profit when pricing corrects back to equilibrium. The index fund trade hedges the stock position. Speed is critical to act before passive funds finish rebalancing.
Additional chances come around quarterly earnings events. Stocks dropping out of an index see selling pressure as funds remove positions. HFT firms buy the undervalued shares and sell short corresponding ETFs to capture spreads. Aside from scheduled events, corporate actions like spin-offs, mergers, IPOs, and special dividends also cause temporary dislocations.
Arbitrageurs monitor index rules and quickly detect coming weight changes using statistical models, machine learning, and natural language processing. Acting fast allows them to trade ahead of passive flows. Opportunities also exist in fixed-income, commodity, and currency-hedged ETFs when pricing diverges from NAV.
Low latency feeds and co-located infrastructure provide the speed to identify and act on arb trades before spreads normalize. Even split-second advantages make a difference when spreads are small. While adding liquidity around events, regulators watch for manipulation. Arbitrage should be grounded in models rather than moving markets.
Index arbitrage is a valuable tool for enabling passive funds to operate efficiently when used properly. HFT helps narrow significant short-term disparities through sophisticated models and microsecond speed. The strategy provides liquidity, but oversight ensures market integrity is maintained. Index arb relies on detecting and quickly trading temporary ETF pricing inefficiencies.
6. News-based trading
News-based trading seeks to capitalize on significant announcements that impact asset prices before human traders react. Algorithms ingest and analyze news feeds, earnings releases, regulatory filings, social media, and other text sources to identify tradable events using natural language processing and machine learning. Logic is preprogrammed to trade based on keywords, semantics, sentiment shifts, and historical data to predict price impacts.
Earnings surprises, merger announcements, product launches, FDA rulings, executive changes, and macroeconomic data releases offer trading opportunities. Preprogrammed logic reacts to events faster than human perception allows, facilitated by low-latency market data feeds and co-located servers. Neural networks analyze text and convert it into actionable trading signals. More advanced AI links related assets based on historical patterns.
Sometimes, strategies assume announcements will cause short-term momentum in a predictable direction. Others use more sophisticated analytical models to estimate likely price and volatility impacts. For scheduled events, algorithms monitor flows and positioning for pre-release cues suggesting surprise direction.
Information leakage provides an edge, with machine learning detecting early price action in futures, currencies, and ETFs, implying upcoming data surprises. Text analytics sometimes uncover numbers or keywords from newswires milliseconds before headlines. Direct data feeds are faster than aggregated summaries.
Systems filter the firehose of information flow to focus only on material events with tradable outcomes. Not all news is actionable. Looking forward, AI and alternative data sources like social media sentiment, web traffic, and satellite imagery sometimes reveal even more signals ahead of news events.
7. Low-latency trading
Low latency trading aims to exploit short-term pricing inefficiencies and arbitrage opportunities by executing at the fastest possible speeds. Even small improvements in system speeds allow HFT firms to act before competitors in a market where milliseconds matter. Strategies take advantage of brief pricing discrepancies between assets and exchanges by trading large volumes to maximize cumulative profits.
Speed advantages allow low latency systems to detect block trades and dark pool activity to trade ahead of coming price impacts. Speed also enhances market-making and statistical arbitrage strategies through improved queue position and fill rates. Winning by milliseconds requires minimized technical latency through direct data feeds, co-located servers, and short network routing.
On the hardware side, FPGAs, GPUs, and parallel processing accelerate data analysis and order generation. Code is optimized by removing unnecessary functions that create delay. The focus is on processing velocity rather than complexity. Latency arbitrage is ultimately a technological arms race to shave off microseconds.
While faster trading offers liquidity, arguments exist on appropriate speed limits and controls to prevent manipulation. Regulators monitor for destabilizing volatility across fragmented venues. Low latency brings markets closer to efficiency, but oversight ensures stability and fairness.
Looking forward, AI and quantum computing might react in nanoseconds based on learning rather than predefined logic. However, regulators will also need to evolve oversight alongside these technologies. HFT provides benefits like liquidity and price discovery but requires proper safeguards against exploitation.
High-frequency trading strategies leverage speed and quantitative modeling to capitalize on short-term inefficiencies, providing liquidity but requiring oversight to ensure market integrity.
What is the average return on HFT?
The exact average return on HFT is difficult to pinpoint, as HFT firms generally keep their detailed trading strategies and performance metrics private. However, most estimates put the average yearly return from HFT strategies between 5-15%, with the top firms generating returns of 20% or more in good years. These returns come almost entirely from exploiting minor pricing inefficiencies and arbitrage opportunities rather than from speculating on the marketâs overall direction.
A 2010 study by Brogaard found that HFT activity provided an estimated trading profit of Rs 24,800 crore per year for the entire HFT industry. Another study by Narang in 2009 estimated the average daily HFT profit to be Rs 1,512 crore across the industry. Assuming 252 trading days per year, that would equate to over Rs 3,81,000 crore in yearly profits across HFT firms.
These industry-wide profit estimates translate to substantial returns when considering the amount of trading capital deployed by HFT firms. Top HFT firms sometimes trade with portfolios in the hundreds of crores or low thousands of crores. Assuming a firm trades Rs 7,000 crore in capital and generates Rs 700 crore in yearly profit, that would represent a 10% average annual return purely from HFT strategies.
However, HFT returns fluctuate widely from year to year based on market conditions. Periods of volatility and diverging prices across exchanges offer the most profit potential for HFT arbitrage strategies. But calm, low-volatility markets offer fewer exploitable inefficiencies. HFT returns above 20% are possible in active, volatile markets but are able to dip close to zero in quiet markets.
In addition, HFT returns have declined over the years as the strategy has become more widespread and competitive. The returns were frequently exceptionally high in the early 2000s, sometimes exceeding 100% yearly when HFT was less used. However, as more firms have adopted HFT systems, exploitable inefficiencies get arbitraged away much more quickly, reducing the potential profits for all firms.
Regulatory changes have also impacted HFT returns. For example, the introduction of Regulation NMS in 2007 led to an explosion in HFT growth for a few years; however, later regulations, such as the elimination of flash orders and restrictions on colocation services, have limited profit opportunities and have brought average HFT returns down.
How do HFT traders make money?
High-frequency traders (HFTs) make money by using sophisticated algorithms running on powerful computers to transact large orders at ultra-fast speeds measured in milliseconds or microseconds. By trading in high volumes and capitalizing on tiny discrepancies in prices across markets, HFTs are able to accumulate small, low-risk profits that add up over time.
The main high-frequency trading (HFT) strategies include market making, where HFTs capture small profits from high volumes of trades by quickly posting and canceling limit orders at the bid and ask prices; arbitrage, which capitalizes on small price differences for the same assets on multiple exchanges by simultaneously buying low and selling high; structural strategies that detect pending large orders based on subtle patterns and then trade ahead of them; and directional strategies that leverage speed advantages to predict and trade just before price moves by mining data for clues about impending order imbalances or price changes milliseconds before they occur.
Other common HFT strategies include latency arbitrage, liquidity detection, quote stuffing, spoofing, and momentum ignition. Latency arbitrage exploits speed advantages to profit from price changes that occur on certain exchanges fractions of a second before others. Liquidity detection involves discovering hidden pockets of liquidity and trading against them. Quote stuffing and spoofing involve manipulating order flow to create a false sense of supply or demand to influence prices. Momentum ignition aims to initiate rapid price moves through high-volume trading.
To implement these strategies profitably at high speeds, HFT systems require expensive, specialized hardware like GPUs, FPGAs or ASICs, colocation services, and ultra-low latency networks. Fibre optic routes between exchanges in New Jersey and Chicago shave vital milliseconds off trading times. Co-locating company servers directly next to an exchangeâs matching engines provides microsecond latency advantages.
The high costs of HFT infrastructure pose barriers to entry but allow successful HFT firms to scale strategies across massive trade volumes. Top HFT firms execute over 5 million trades per day. Even profits as low as a fraction of a rupee per share traded stack up to over Rs 7,000 crore in annual profits for leading HFT companies.
How accurate is HFT?
The accuracy of high-frequency trading strategies is extremely high, with the best systems achieving over 99% accuracy on trades. This level of precision is made possible by advanced machine learning algorithms and powerful computing hardware that analyze markets and execute orders in nanoseconds.
HFT systems rely on complex predictive models that identify temporary pricing anomalies and market inefficiencies. The models are trained on vast historical datasets of ticks, time & sales, order book snapshots, and other market data. Algorithms ingest this data and continuously optimize massive numbers of parameters to detect patterns invisible to humans.
The best HFT algorithms are highly adaptive, monitoring their trading outcomes in real-time and constantly updating their logic to improve profitability. Over time, they learn which signals and strategies work best under different market conditions. This iterative optimization process leads to extremely accurate systems.
Additionally, HFT firms exert great effort to minimize technical errors and flawed order execution. Their trading infrastructure is engineered for speed, determinism, and precision. Strategies are back-tested extensively before live deployment to weed out undesirable behaviors. Firms meticulously monitor system performance and trading results to identify any potential bugs or degraded accuracy.
This rigorous approach results in negligible rates of technical errors or mistakes for most HFT systems. The accuracy of trade timing and execution is especially critical. Large orders are broken down programmatically into precise sequences of smaller orders to avoid tipping off the market. Sophisticated execution algorithms time each slice to manage market impact and ensure full-fill rates near 100%.
Of course, even with near-perfect technical accuracy, the predictive accuracy of the underlying algorithms has limits. No model is able to foresee all market movements, and even the most advanced quantitative strategies cannot completely account for human psychology and shifting investor sentiment. Major shocks and volatility degrade short-term performance.
To mitigate losses during unpredictable swings, HFT systems incorporate tight risk controls. Individual position sizes are kept small, and dynamic stop-loss orders liquidate losing trades before losses escalate. By distributing risk across thousands of simultaneous positions and maintaining low exposure, algorithms achieve strong risk-adjusted returns even if predicting the market direction wrongly on occasion.
What are the advantages of HFT?
The advantages offered by HFT are improved liquidity, narrowed bid-ask spreads, enhanced price discovery and market efficiency, reduced short-term volatility, lowered transaction costs, economies of scale in trading infrastructure, automation and mathematization on the buy side, financial technology innovations, human capital development, and tax revenue.
One major advantage of HFT is improved liquidity. HFT market-making strategies involve continuously posting and updating limit orders to buy and sell. This greatly increases the âdepth of the book,â meaning more shares available at each bid or ask price. Greater liquidity facilitates larger trades from institutional investors without significant price impact.
Relatedly, bid-ask spreads are narrowed through HFT competition. Wholesale market makers provide two-sided quotes for both buying and selling. HFTs competing for market-making business drives spread down to fractions of a rupee, reducing costs for other investors. Spreads on highly liquid stocks have fallen over 80% since the rise of HFT.
HFT enhances price discovery and market efficiency. Their algorithms react within microseconds to new data or price changes on related assets. This rapidly incorporates new information into prices before most human traders even blink. As a result, prices more closely reflect true equilibrium values informed by current conditions.
HFT also reduces short-term volatility by supplying liquidity during turbulent periods. While long-term investors sometimes exit positions and withdraw from the market during turmoil, HFT systems typically operate non-stop with fixed risk parameters. Their continuous quoting activity calms volatile swings and mitigates price dislocations.
Furthermore, transaction costs are lowered through fragmentation reduction strategies. HFT arbitrage across the hundreds of exchanges, dark pools, and electronic communication networks (ECNs) enforces unified pricing. This saves money for institutional investors by allowing them to execute larger orders in pieces across venues without price divergence.
The prevalence of HFT also creates economies of scale in trading infrastructure. Exchanges must invest heavily in speed enhancements like colocation services and faster network routing to stay competitive. However, this reduces latency and increases capacity for all participants, not just HFT firms. The costs are amortized across the industry.
Additionally, HFT brings mathematization and automation to the buy side. Sophisticated algorithms allow quantitative hedge funds and other institutional investors to implement complex multi-asset trading strategies beyond just equities. This provides diversification, risk management, and other advantages to investment funds employing HFT systems.
The level of software engineering expertise required for HFT also spurs technology innovations that benefit the broader finance industry. Blockchain, in-memory databases, machine learning, and other technologies were pioneered by HFT firms and later applied more widely. Technology jobs and skills training centered around HFT improve human capital in the financial sector.
Responsible HFT adhering to ethical practices contributes to tax revenue. Though often criticized for an unfair advantage, profitable HFT firms do pay significant taxes that fund government services. Estimates suggest nearly âš7,000 crore in annual state and local tax revenues from HFT in India.
What are the limitations of HFT?
High-frequency trading also has inherent limitations like the ultra-short timeframe, need for speed, and purely quantitative nature of HFT, which lead to key constraints around volatility, market impact, costs, and adaptability.
The extremely short time horizon of HFT algorithms, holding positions for milliseconds or less, makes them vulnerable to sudden volatility. Most HFT strategies assume normal market conditions and relatively stable correlations. Significantly heightened volatility or a breakdown in historical relationships causes models to fail. HFT firms sometimes lose substantial capital very quickly during periods of market stress like the 2010 Flash Crash.
Relatedly, the market impact from high HFT volumes exacerbates volatility spikes. Since HFT systems react similarly to price movements, their collective reaction reinforces the original move even further. This self-perpetuating feedback loop leads to outsized swings as machines rapidly amplify each otherâs behaviors. Humans must intervene to break the cycle.
HFT also cannot execute more sophisticated, longer-term trading strategies beyond arbitrage and market making. Strategies based on fundamental valuation, technical chart patterns, macroeconomic analysis, and other factors require human insight and oversight. This precludes HFT funds from benefiting from proven investing approaches.
The dependence on obtaining and reacting to market data faster than competitors leads to diminishing returns in speed investment. Gaining microseconds of advantage requires exponential technological spending on the fastest hardware, data lines, and network proximity services. However, the profits realized from such infinitesimal speed gains decrease proportionally.
Costs also accrue from running complex HFT infrastructure virtually non-stop. Keeping data centers staffed and maintained around the clock, servers powered on perpetually, and connectivity robust enough to avoid any downtime or latency costs millions. These expenses act as a tax on the HFT approach.
Purely quantitative models have difficulty incorporating qualitative factors like earnings call commentary, management shake-ups, product launches, strategic shifts, and geopolitical events. But these human-centric inputs often drive stock prices. Unable to assess softer information, HFT algorithms miss trading catalysts.
HFT systems also struggle to adjust algorithmic logic to shifting market conditions. Human traders intuit when markets transition into new regimes requiring updated strategies. However, HFT algorithms rely on patterns inferred from historical data that grow stale. New market paradigms render existing models ineffective.
Frequent software updates and retraining models on recent data help HFT systems adapt. However, this process lags behind human traders augmented with judgment, intuition, and inductive reasoning. Markets evolve sometimes faster than algorithms are updated.
There are also inherent transaction costs from the huge volume of trades HFT generates despite the low cost per trade. Exchanges and electronic communication networks charge fees that add up when trading billions of shares per month. These costs affect the profitability threshold for viable trades.
What are the regulations on HFT?
In India, high-frequency trading (HFT) and algorithmic trading are regulated by the Securities and Exchange Board of India (SEBI). SEBI first introduced regulations related to algorithmic trading in March 2008, which required that all algorithmic orders be tagged with a unique ID number. In March 2009, SEBI proposed new guidelines for algorithmic trading, which required algorithmic traders to have sufficient risk management controls and systems in place. The guidelines prohibited self-trades by brokers and required that brokers provide safety features like price bands, quantity limits, and automatic cancellation of orders.
In April 2010, SEBI notified regulations related to direct market access, colocation, and algorithmic trading. These regulations required that algorithmic traders obtain prior permission from exchanges, put in place system audit trails, and have proper security features. In September 2011, SEBI issued guidelines on minimum tick size, randomization of orders, and synchronization of trade engines across exchanges. These were intended to minimize manipulative strategies like order stuffing and layering in HFT.
In March 2012, SEBI empowered stock exchanges to penalize algorithmic traders for any unfair trade practices. Exchanges were allowed to take immediate action against errant algorithmic traders. SEBI also specified guidelines on testing, use of kill switches, etc., for algorithmic trading systems. The regulator continues to refine regulations to promote the orderly functioning of algorithmic trading in India.
Internationally, regulators have taken diverse approaches to regulating HFT. In the United States, the Securities and Exchange Commission (SEC) and the Commodity Futures Trading Commission (CFTC) first focused on equity market microstructure issues like colocation and order types. In 2009, flash orders that gave HFTs an advantage were banned. However, the May 2010 flash crash triggered a broader review.
In April 2011, IOSCO released recommendations on algorithmic trading, including pre-trade controls, circuit breakers, monitoring, and compliance. In March 2012, the CFTC implemented registration rules for HFTs. The SECâs Regulation Systems Compliance and Integrity (Reg SCI) introduced stricter technology monitoring rules in 2015. The EUâs Markets in Financial Instruments Directive (MiFID II), effective 2018, mandates detailed reporting by HFTs and stringent testing of algorithms.
In Asia, Japan requires HFT firms to register with the Financial Services Agency and submit monthly reports. South Korea introduced guidelines in 2010 requiring real-time monitoring of algorithms by exchanges. Singapore, Hong Kong, and Australia have also enhanced supervision of HFT in recent years. Monitoring of algo orders, kill switches, minimum resting times, etc., is common across jurisdictions. However, specific regulations continue to evolve with the nature of HFT strategies and technologies.
What are some controversies about HFT?
One major controversy is around the fairness of HFT and whether it gives high-frequency traders an unfair advantage over other market participants. The speed advantage enables HFT firms to detect trading patterns and place orders microseconds before others. Critics argue that this amounts to front-running, even if it is technically legal. Flash crashes triggered by HFTs also undermine overall market confidence. However, proponents counter that HFT provides crucial liquidity and narrows spreads for all investors. The core issues are around unequal access and whether blindingly fast trading distorts market quality.
Related to this is the controversy around preferential access to trading venues through colocation services and customized data feeds. Exchanges sell colocation space and proprietary data feeds that allow HFT firms to reduce latency and gain valuable speed advantages. This raises concerns about two-tiered access to public markets and skewed competition. While exchanges argue that they are selling services equally to all participants, critics point out that it entrenches the position of dominant HFT firms. There are also fears that retail investors will suffer due to HFT activity.
Another major controversy is the lack of transparency about HFT activities to regulators and the public. HFT firms closely guard their algorithms and trading strategies. The âblack boxâ nature makes it difficult to analyze their market impact. Opacity also enables unfair practices to go undetected. However, mandatory disclosures could expose valuable IP to competitors. Striking the right balance between transparency and protecting proprietary IP has been tricky.
The phenomenon of âspoofingâ and âlayeringâ by HFTs has drawn scrutiny. This involves submitting fake orders to influence market prices and then capitalizing on the subsequent movements. Critics view it as pure manipulation, though proving intent is difficult. There are also concerns about âquote stuffing,â where huge volumes of orders are sent to slow the market and create arbitrage opportunities for HFTs.
The perceived proliferation of manipulative and destabilizing HFT strategies has fueled calls for a financial transactions tax to curb excessive speculation. However, this is opposed by the industry as being infeasible or damaging to liquidity. Wider concerns about computerized trading increasing systemic risks are another simmering worry among regulators. However, there is little consensus on balancing innovation and stability through HFT regulation.
What is the future of HFT?
As technology continues to advance, HFT firms will likely gain more speed and sophistication in their trading algorithms and infrastructure, though increased regulation sometimes limits HFT strategies and participation if deemed unfair or disruptive to market stability and integrity.
One ongoing trend that is likely to continue is the arms race for speed. HFT firms are constantly seeking to gain millisecond advantages over competitors by investing in faster hardware and connections. This includes efforts like co-locating servers directly at exchange data centers to minimize latency. As technology improves, the speeds of trading will keep increasing. For example, the adoption of 5G networks could allow near-instantaneous wireless trading speeds. However, diminishing returns sometimes eventually set in â while microseconds provide an edge today, nanoseconds in the future sometimes do not yield meaningful advantages. Regulators sometimes also step in if pursuits of marginal speed benefits are seen as destabilizing. But the quest for speed does not appear to be dying down yet.
HFT is also expected to expand across more asset classes and into new markets. While most prevalent in equities, HFT has moved into currencies, futures, and other assets. New exchange-traded products like cryptocurrencies are also seeing HFT penetration. As technology becomes more ubiquitous globally, HFT will spread into emerging markets. However, differences in market microstructure, regulation, infrastructure, and other factors across regions constrain HFT capabilities. Firms will need to adapt strategies to suit each marketâs unique characteristics.
The use of increasingly sophisticated algorithms is set to rise. HFT firms deploy a range of algorithms optimized for different strategies â such as market making, arbitrage, momentum trading, etc. Machine learning and artificial intelligence are being incorporated to develop âadaptiveâ algorithms that self-learn, evolve with changing market conditions, and execute more complex trades. While AI holds promise, over-reliance heightens systemic risks if algorithms behave unpredictably during periods of stress. Firms will need rigorous testing and risk controls as AI usage intensifies.
Another trend is the automation of trading processes from start to finish. This includes algorithmic development, strategy design, pre-trade analysis, trade execution, post-trade processing, and risk management. Full automation enables HFT to scale dramatically while minimizing humans in the loop. However, automating too much could also increase the consequences of algo âflash crashes.â Finding the right balance will help avoid catastrophic failures.
Cloud computing is also gaining traction among HFT firms to carry out computationally intensive tasks faster while minimizing hardware investments. As security improves, cloud-based processing offers cost efficiencies at scale. However, migrating to third-party cloud servers also entails privacy risks and reduced control. Regulatory comfort with widespread cloud usage in finance remains limited. Until data security and sovereignty concerns are addressed, cloud adoption by HFT will be gradual.
Looking ahead as HFT grows more pervasive, calls for safeguards against volatility and disruption are rising globally. Strict âspeed bumpsâ could be imposed to curb excessive transactions. Taxes targeting HFT specifically could be introduced. However, any policy actions should weigh benefits against costs to avoid over-regulation. The objective should be optimizing stability while encouraging financial innovation. A collaborative approach between regulators and industry helps ensure that HFT remains a constructive force.
How do I become a HFT trader?
To become a high-frequency trader, first obtain an educational background in computer science, mathematics, or engineering to develop the technical skills needed to design and implement automated trading systems. Then, I will seek out job experience at proprietary trading firms or hedge funds that specialize in high-frequency trading strategies to gain practical knowledge of financial markets and sophisticated trading algorithms. Becoming a successful HFT trader takes dedication, technical skills, and substantial capital.
A quantitative background is essential for HFT. Degrees in fields like computer science, engineering, mathematics, statistics, or finance provide relevant hard skills. Coursework in programming, machine learning, algorithms, and data analysis is especially useful. Options include a bachelorâs degree, masterâs degree, or PhD. Academic credentials from top universities demonstrate analytical rigor to potential HFT employers. Supplement formal education by teaching yourself skills like Python coding.
Learn the intricacies of markets in which you aim to deploy HFT â like equities, futures, FX, or derivatives. Understand factors driving liquidity, volatility, asset correlations, and other dynamics. Familiarise yourself with exchanges, regulations, structures, and instruments. Knowledge of market microstructure is vital to recognize opportunities and avoid pitfalls. Read books, publications, forums, and news covering your target markets. Experience through internships or junior trading roles builds first-hand expertise.
Nothing builds HFT expertise as effectively as real-world trading experience. Start with paper trading strategies before allocating capital. Open a personal trading account to practice implementing ideas in the live market. Join proprietary trading firms or arcades to utilize their capital and infrastructure in exchange for splitting profits. Progress to funded accounts as you gain more experience. Working at established HFT firms mentors you in their strategies and systems. Eventually, you can become fully independent with your own capital once you are seasoned.
HFT algorithms unravel quickly in volatile or illiquid conditions. Program defensively to limit downside, particularly during market disruptions that will occur. Stress test systems and set stops to contain potential losses on errant trades. Ensure you have the technology infrastructure to monitor risk in real-time across portfolios. Refine controls as you encounter new scenarios. Risk management separates successful stock market traders from gamblers. Survivability is key.
What is the highest-paid HFT?
Among firms, the highest earners in Indian stock markets tend to be secretive proprietary trading shops and hedge funds that pioneer the latest HFT strategies, like Mumbai-based AlphaGrep Securities and Bangaloreâs Chanakya HFT.
AlphaGrep Securities was estimated to earn over Rs 700 crore in trading revenue in 2020. It has become an HFT juggernaut with over 100 employees across offices in Mumbai, Delhi, and Bangalore. AlphaGrep deploys artificial intelligence and machine learning to implement complex data-driven trading strategies across assets ranging from equities to currencies.
Chanakya HFT has also established itself as one of the largest and most successful HFT players in India. Though private, Chanakya discloses limited financial information as it is not required to separate HFT results from other operations. However, estimates indicate Chanakya likely generates over Rs 500 crore annually from its HFT and market-making activities. The company actively trades on NSE, BSE, and MCX using smart order routing and proprietary execution algorithms.
How much does HFT cost?
The costs of building and operating HFT systems run into crores of rupees. The high speed and complex infrastructure required to engage in HFT make it an extremely capital-intensive strategy. The computer hardware and connectivity needed to execute trades in microseconds is enormously expensive. HFT firms invest heavily in powerful servers, CPUs, GPUs, and networking gear tailored for speed. Ongoing upgrades to maintain speed advantages cost crores per year. Co-locating servers in the same premises as exchanges allow for reducing latency but add huge rent and data feed costs. The fastest connections using microwave/laser technology between key hubs like Mumbai and Delhi reportedly cost over Rs 140 crore per link.
HFT strategies require complex statistical algorithms coded by top programmers. Recruiting and retaining quantitative experts and developers drives up compensation costs. Specialized commercial software for trading, risk management, and surveillance also entails licensing expenses. Firms further spend heavily on building machine learning capabilities. Monitoring all systems in real-time for both functionality and security requires significant personnel.
To capitalize on market inefficiencies, HFT strategies depend on live data feeds from exchanges. These include level 1, level 2, total view, and proprietary feeds, which are vital but expensive. A single exchange feed sometimes costs over Rs 1 lakh per month. Firms subscribe to feeds across numerous exchanges, asset classes, and geographies, which adds up quickly. Paper trading strategies first before subscribing also incur data costs.
Is high-frequency trading profitable?
Yes, high-frequency trading is profitable at times. HFT has become a major force in equity markets due to its substantial profit potential from small, repetitive trades executed at blazing speeds. However, as competition intensifies and regulators intervene, the profitability of HFT has come under pressure in recent years.
In its early years, HFT was extremely profitable, allowing firms to gain market share rapidly. By trading ahead of slower investors, HFT firms could benefit from price movements caused by major orders. Their algorithms were also better at finding liquidity and minimizing trading costs. This allowed HFT firms to capture significant profits as financial markets went electronic.
Studies found that in 2009-2010, the top HFT firms earned over Rs 35,000 crore in profits annually. Over 95% of all orders placed on electronic exchanges were high-frequency algorithmic trades. Trading venues also provided rebates and incentives to liquidity-providing HFT firms, boosting their profitability. The combination of speed, small spreads, volume incentives, and low commission fees created ideal conditions.
However, in recent years, HFTâs profit margins have declined significantly. From peak pre-tax profit margins of over 60% between 2007-2009, margins dropped to around 10-20% by 2019. Competition has intensified as HFT strategies have proliferated, cutting into market share. HFT firms fiercely compete to implement new algorithms microseconds faster than rivals. Strategies get commoditized quickly, leaving little time to benefit. Exodus of talent has also increased with programmers moving to hedge funds or starting their own shops after gaining expertise.
Exchanges and regulators have made moves to curb predatory HFT activity. Practices like spoofing, layering, quote stuffing, etc., have faced crackdowns. Exchanges have reduced maker-taker rebates and widened tick sizes to reduce gaming. SEBIâs new algorithmic trading rules and reforms after the NSEL crash have also added checks on HFT in India. These regulations have constrained profits.
HFT still remains profitable for top players like Chanakya HFT and AlphaGrep Securities, which have institutionalized knowledge and capabilities in India. The largest domestic HFT firms continue making around Rs 700 â Rs 2100 crore in annual profits supported by their technology edge. Massive scale across Indian equities, derivatives, and currency markets aids their profits.
Profits also vary drastically between strategies and asset classes. Low latency arbitrage and market-making in liquid instruments like index ETFs remain lucrative. However, strategies beyond core markets are less consistent. HFT in assets like bonds, futures, and currencies faces limitations. Geographic nuances across markets also impact strategy effectiveness.
Is HFT trading illegal?
High-frequency trading itself is entirely legal and permissible under securities laws and regulations. However, certain unethical practices associated with HFT have come under scrutiny from regulators in recent years.
HFT firms act as liquidity providers similar to traditional market makers. By posting simultaneous buy and sell orders, they facilitate orderly markets and tighter spreads, benefiting all investors. Their huge transaction volumes and razor-thin margins carry out legitimate market-making functions.
Is HFT the same as flash trading?
High-frequency trading and flash trading are not identical. HFT refers broadly to fully automated, algorithmic trading done at extremely high speeds, typically using co-located infrastructure for minimizing latency. It encompasses strategies executed multiple times per second across markets and assets. Flash trading specifically indicates seeing buy or sell orders before the wider market and exploiting this visibility advantage to trade ahead for profits. While certain HFT firms sometimes engage in flash trading, it is not intrinsic to HFT itself. Rather, flash trading represents a particular controversial practice that high-frequency traders sometimes employ along with other strategies. However, flash trading on unfair informational asymmetries falls into a grey area legally and ethically.
Leave a Reply
Recently Published
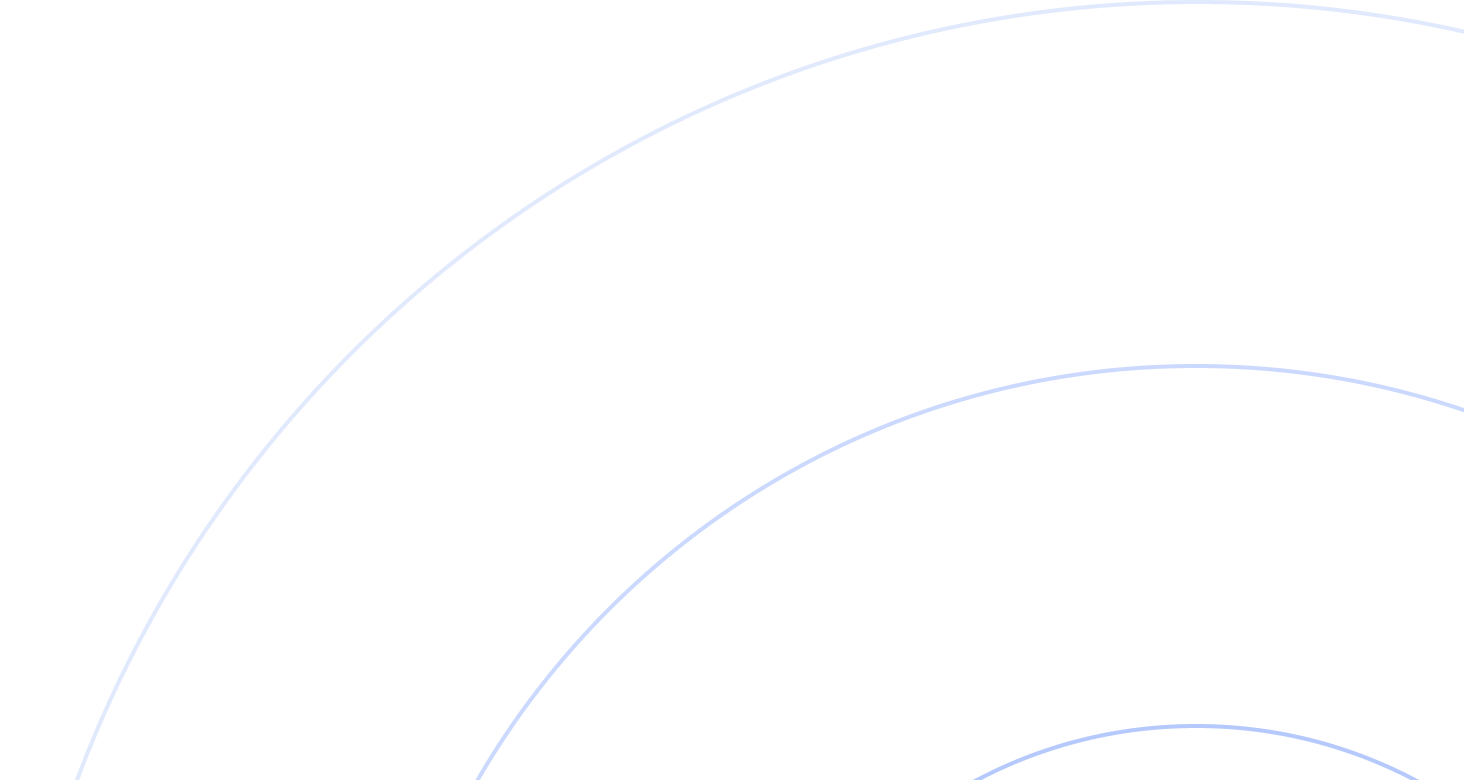
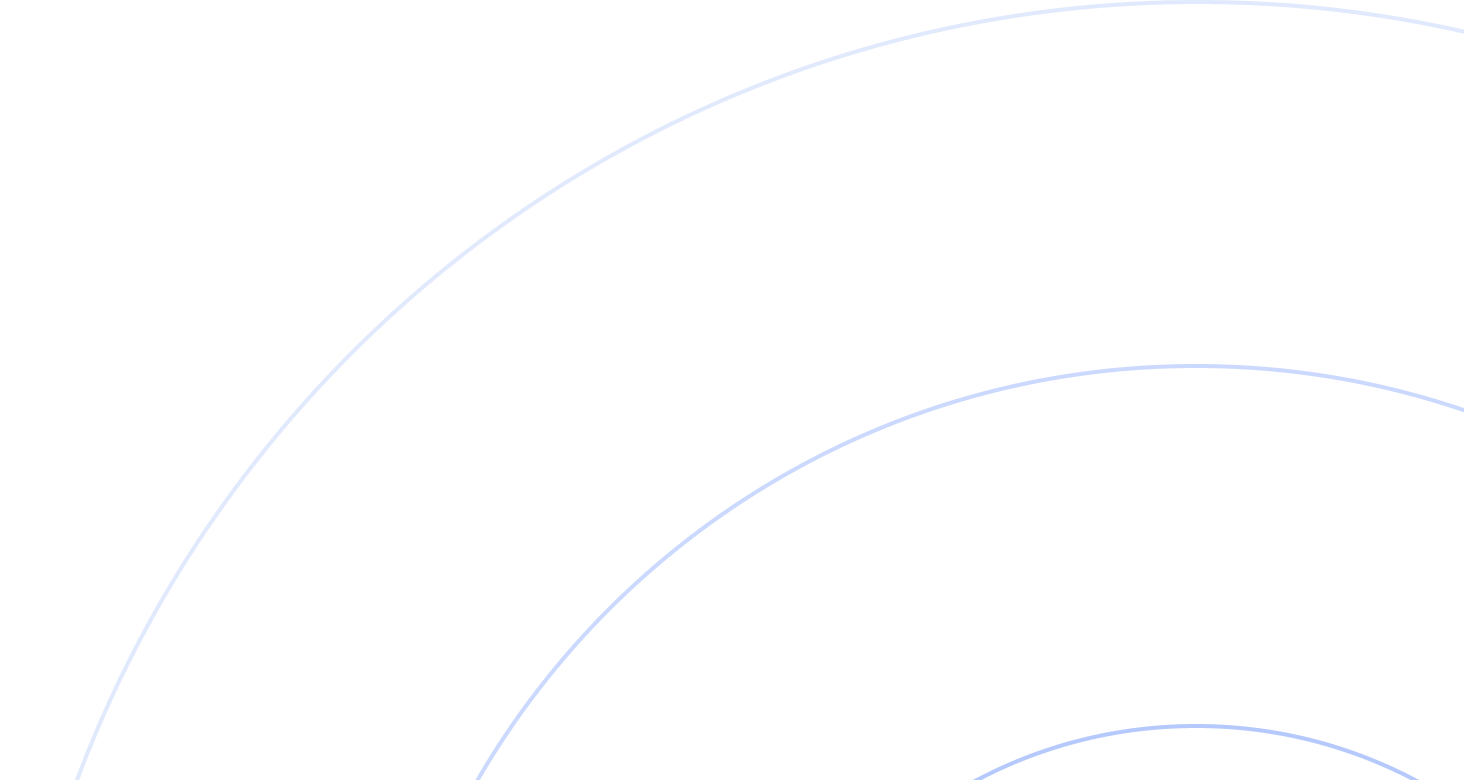
Join the stock market revolution.
Get ahead of the learning curve, with knowledge delivered straight to your inbox. No spam, we keep it simple.
Comments